Manual searching for legal basis such as paragraphs, articles, and laws when preparing for a tax court hearing is time-consuming. In this paper, we use extreme multilabel text classification approach to predict paragraphs, articles, and laws relevant to an appeal on the Indonesian Tax Court Ruling documents. Traditional machine learning methods, such as random forest, can produce a good performance for an extreme multilabel text classification problem but requires training a huge number of separate classifiers. Meanwhile, deep learning methods such as convolutional neural networks (CNN) can effectively solve the extreme multilabel text classification problem. Furthermore, the use of IndoBERT embedding to represent Indonesian text in multilabel classification problems has not been explored much. This research proposes a single channel CNN model with IndoBERT embedding to solve extreme multilabel text classification problems on Indonesian Tax Court Ruling documents. We use three labeling scenarios: paragraph-level label scenario, article-level label scenario, and law-level label scenario. Our experiments demonstrate that our proposed model (CNN+IndoBERT) outperforms the single channel CNN with Word2Vec embedding and the single channel CNN with fastText embedding in all three labeling scenarios. In addition, our model also outperforms the multiple channel CNN with IndoBERT embedding in both paragraph and article-level label scenarios.
Read full-text article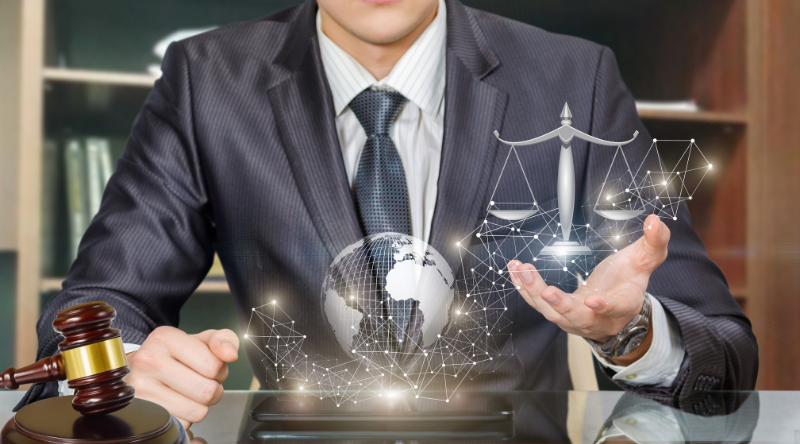